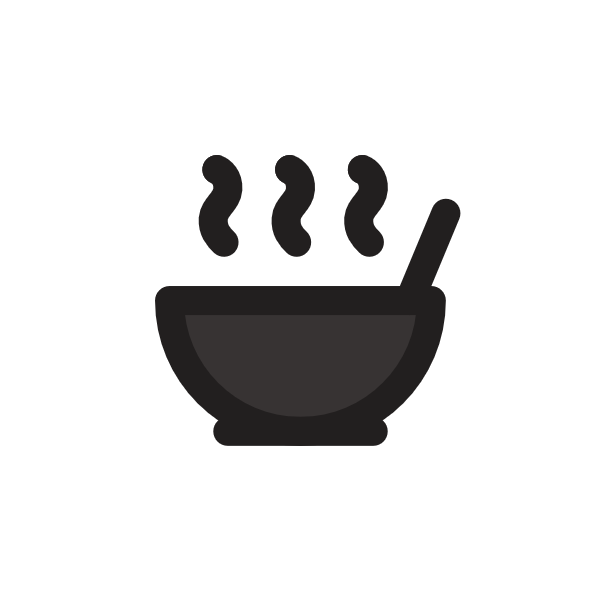
Cocojunk
🚀 Dive deep with CocoJunk – your destination for detailed, well-researched articles across science, technology, culture, and more. Explore knowledge that matters, explained in plain English.
Selection bias
Read the original article here.
Okay, here is the detailed educational resource on Selection Bias, reframed within the context of "Digital Manipulation: How They Use Data to Control You".
Understanding Selection Bias in the Age of Digital Data
In the digital age, data is power. It fuels everything from the algorithms that recommend your next video to watch, to the targeted ads you see, to the political messages designed to influence your vote. But what happens when the data collected isn't a true reflection of reality? This is where selection bias becomes a critical concept, particularly in understanding how digital systems can inadvertently (or sometimes deliberately) distort our understanding, limit our choices, and even exert control.
Selection bias is a fundamental flaw in data collection and analysis that can lead to misleading conclusions. When data is used to make decisions about individuals or groups, a biased sample means those decisions are based on an inaccurate picture of the world, potentially enabling manipulation or unfair treatment.
What is Selection Bias?
Selection bias occurs when the way data is collected leads to a sample that is not representative of the larger population or phenomenon being studied.
Selection Bias: The distortion introduced into a statistical analysis or conclusion when the individuals, groups, or data points chosen for analysis are selected in a way that is not properly random, causing the sample to be unrepresentative of the target population. This systematic error can lead to false conclusions.
Think of it like trying to understand the dietary habits of an entire city by only surveying people leaving a vegetarian restaurant. Your data sample (people leaving the vegetarian restaurant) is highly unlikely to represent the diverse eating habits of the city's total population. In the digital world, this problem is pervasive, often hidden behind complex algorithms and vast datasets.
The Problem of Unrepresentative Samples in Digital Systems
Digital platforms and data collectors gather enormous amounts of information. However, this data often comes from specific user groups or is collected under particular circumstances, creating inherent biases. When algorithms or decisions are built upon this biased data, they perpetuate and even amplify the distortions present in the original sample. This can limit your exposure to information, steer your behavior, or make unfair assumptions about you based on data that doesn't truly reflect you or the wider world.
Types of Selection Bias and Their Digital Manifestations
Selection bias isn't a single issue but rather a category encompassing various ways data can become unrepresentative. Here are common types, illustrated with examples relevant to digital manipulation and data control:
1. Sampling Bias
Sampling bias is the most direct form of selection bias, occurring when the method of selecting participants or data points means some members of the target population are less likely to be included than others.
Sampling Bias: A systematic error introduced by a non-random method of sampling a population, resulting in a biased sample where participants or data points are not equally balanced or objectively represented.
While sometimes discussed separately, sampling bias is often considered a subtype of selection bias. A key distinction sometimes made is that sampling bias affects external validity (can the results be generalized to the whole population?), while selection bias can affect internal validity (are the relationships observed within the sample accurate?). In digital contexts, both are critical because biased samples lead to models and systems that perform poorly or unfairly when applied to the real, diverse population.
Digital Examples & Explanations:
- Online Surveys: Data collected from voluntary online surveys is highly susceptible to sampling bias. The sample only includes users who:
- Saw the survey.
- Use the platform where the survey is hosted.
- Chose to participate (see Volunteer Bias below).
- Have the time and ability to complete it. This sample is unlikely to represent the general public or even the platform's entire user base. Decisions based on such survey data (e.g., product features, content strategies) might only cater to a vocal minority.
- Platform-Specific Data: Analyzing user behavior solely on one social media platform tells you about users of that platform, not necessarily the broader internet population or even demographics who avoid that platform. Policies or content strategies based on this data might fail elsewhere or disadvantage groups not present on the platform.
- Device/Browser Bias: Data collected primarily from users of a specific operating system, browser, or device type (e.g., mobile app users vs. desktop web users) will not represent the experiences or behaviors of users on other platforms. This can lead to unequal user experiences or biased feature rollouts.
- Excluding Non-Users: Training AI models or understanding market trends solely on data from existing users ignores the characteristics and reasons of people who don't use the product or service. This makes it hard to attract new users or understand barriers to adoption.
2. Time Interval Bias
This bias occurs when the data collection or analysis period is chosen or terminated in a way that skews the results, often to support a desired outcome.
Digital Examples & Explanations:
- Early Termination of A/B Tests: Companies frequently use A/B testing to compare two versions of a webpage, ad, or feature. Terminating a test early because one version shows a statistically significant positive result at that moment can be misleading. The difference might disappear, reverse, or lead to negative long-term effects (e.g., user burnout) not captured in the short timeframe. This can lead to deploying suboptimal designs based on fleeting data peaks.
- Analyzing Data from Specific Periods: Looking only at user engagement data during a holiday sale might give an inflated view of typical user behavior. Analyzing online political sentiment only right after a major event might not reflect underlying long-term opinions.
3. Exposure Bias (Indication Bias, Susceptibility Bias, Protopathic Bias)
These biases arise when the "exposure" (like seeing an ad, receiving a specific type of content, or being targeted by an algorithm) is not randomly assigned but is dependent on existing characteristics or early symptoms of the individual. This makes it difficult to determine if the exposure caused an outcome, or if the outcome was already more likely due to the pre-existing condition or indication for treatment/exposure.
Indication Bias: A potential confusion between cause and effect when an exposure (like a treatment or targeted content) is given specifically because of an individual's characteristics or risk factors for a particular outcome. This can make the exposure erroneously appear to cause the outcome.
Digital Examples & Explanations:
- Targeted Ads for Vulnerable Groups: If loan companies target ads aggressively at users flagged as having financial difficulties (based on their data profile), and some of these users subsequently take out high-interest loans and face further problems, it might look like the ads caused the financial problems. In reality, the ads were shown to individuals already at higher risk for those problems (the indication for the exposure existed beforehand).
- Content Recommendation: Algorithms learn to recommend content based on past user behavior. If a user has previously shown interest in conspiracy theories (pre-existing susceptibility), the algorithm recommends more similar content. It might appear the algorithm radicalized the user, when it primarily amplified pre-existing tendencies by feeding the susceptibility.
- "Protopathic" Algorithm Flags: If an AI system flags users showing early, subtle signs of distress (e.g., searching certain terms) for intervention or content moderation, and some of those users later exhibit more problematic behavior, the intervention/flagging might seem to have caused the escalation. The bias here is applying the "treatment" (flagging/intervention) after the initial symptoms appeared, potentially missing the true underlying cause.
4. Data Bias (Post-Hoc Selection)
This type involves manipulating or selectively using data after it has been collected, often based on knowing the results or desired outcomes.
Digital Examples & Explanations:
- Cherry-Picking Results: Presenting only the positive case studies for an AI product's performance, while ignoring failures or instances where it caused harm. Companies might showcase glowing testimonials while suppressing negative reviews. This is less pure selection bias and more related to confirmation bias (seeking data that supports a pre-existing belief) and reporting bias (only reporting favorable data), but it relies on the selection of data to present a distorted picture.
- Arbitrarily Excluding Data Points: Removing data from users who behaved unexpectedly ("outliers") without a clear, pre-defined statistical reason. While outlier removal can be valid, doing it solely because a data point hurts a model's performance on average can obscure important edge cases or the experiences of minority groups, leading to models that fail unpredictably in diverse real-world scenarios.
- Analyzing Data Based on Knowing the Outcome: Deciding how to group or analyze user data after seeing preliminary results, to make a specific finding appear stronger. For example, seeing that male users in a certain age range were highly engaged, and then designing the analysis specifically to highlight that group, without having defined this grouping beforehand.
5. Studies/Reporting Bias
While often applied to scientific research, this bias is highly relevant to how digital systems and their impacts are presented to the public and policymakers. It involves the selective reporting or emphasis of findings.
Digital Examples & Explanations:
- Only Publishing Successes: AI research labs, tech companies, or academic researchers may only publish studies showing successful applications or positive impacts of their technology, while failures, negative social consequences, or ethical issues are not reported or downplayed. This creates an overly optimistic and biased view of the technology's readiness and impact.
- Data Dredging (P-Hacking) Presented as Findings: Analyzing a massive dataset for any statistically significant correlations, and then presenting the few significant findings as if they were pre-defined hypotheses that were confirmed. In digital advertising, this could mean trying dozens of ad variations or targeting criteria, finding one combination that performed slightly better by chance, and reporting it as a breakthrough strategy.
- Selective Meta-Analysis: When reviewing the impact of a type of digital intervention (e.g., nudges on a platform, educational apps), a review might selectively include only studies that showed positive effects, ignoring those with null or negative findings.
6. Attrition Bias
This bias occurs due to the loss of participants or data points over time or during a process. If the individuals who drop out, stop using a service, or don't complete a task are systematically different from those who remain, the resulting data will be biased. It's closely related to survivorship bias, where only the "survivors" (those who completed the process or remained in the dataset) are included in the analysis.
Attrition Bias: A type of selection bias caused by the systematic loss of participants or data from a study or dataset over time. If the characteristics of those who drop out or are lost differ significantly from those who remain, the analysis based on the remaining data will be biased.
Digital Examples & Explanations:
- Analyzing User Retention: If a company analyzes data only from users who stay active on their platform, they might misunderstand why users leave. Users who churn might do so due to bugs, poor design, or negative experiences not captured in the data of the "survivors." Product decisions based on this biased data may fail to address the root causes of churn.
- Incomplete User Journeys: Analyzing data only from users who complete a sign-up process, purchase, or onboarding tutorial ignores the data from those who dropped off at various stages. The characteristics and pain points of users who fail to complete the journey are missed.
- Longitudinal Study Dropouts: If a study tracks users' digital habits over months or years, and certain types of users (e.g., those with lower digital literacy, lower income who can't afford consistent access, or those who become disillusioned with the study) are more likely to drop out, the final dataset will not represent the original population.
7. Volunteer Bias (Self-Selection Bias)
This is a specific form of sampling bias where individuals select themselves into a group or study, often because they have a particular interest, motivation, or characteristic that makes them different from the general population.
Digital Examples & Explanations:
- Online Polls & Petitions: Participants are self-selected. People with strong opinions (positive or negative) are much more likely to vote in an online poll or sign a petition than those who are indifferent or unaware. Results from such sources are rarely representative of the general population's views.
- Product Reviews & Ratings: Users who leave reviews are often those who had a particularly good or bad experience, or who are highly motivated to share their opinion. Analyzing only review data will give a skewed picture of average user satisfaction.
- Participants in User Experience Testing/Beta Programs: Individuals who volunteer for these programs may be more tech-savvy, more engaged with the product, or more forgiving of issues than the average user. Feedback from these groups needs to be carefully interpreted.
8. Observer Selection Bias
This philosophical bias, while less common in everyday digital data collection discussions, reminds us that our data is limited by what we are able to observe.
Observer Selection Bias: Bias introduced because the data available for analysis is conditional on the existence or characteristics of the observer (or the system collecting the data). What is observed is filtered by the necessity of someone (or something) being there to observe it.
Digital Examples & Explanations:
- Data Collection Limits: Surveillance systems or data trackers only capture data within their defined scope. Data on activities happening offline, on encrypted platforms the system can't access, or using technologies the system doesn't monitor, is simply unavailable. Decisions or analyses based only on the observable data will miss significant parts of reality.
- Algorithmically Filtered Reality: If an algorithm primarily shows you content similar to what you've engaged with before, your view of the digital world (and even the real world, as reported online) becomes filtered by your past actions and the algorithm's design. You only observe what the system allows or chooses you to observe, leading to filter bubbles and echo chambers where alternative viewpoints or information might be systematically excluded from your observable data stream. This isn't just about your choices (confirmation bias), but about the system selecting what is available for you to observe.
The Consequences: How Biased Data Enables Manipulation and Control
In the context of "Digital Manipulation: How They Use Data to Control You," selection bias isn't just a statistical nuisance; it's a mechanism that can lead to harmful outcomes:
- Algorithmic Bias: AI models trained on biased data will inevitably produce biased outputs. This can lead to unfair loan applications, discriminatory hiring processes, skewed criminal justice predictions, and unequal access to opportunities or information.
- Distorted Reality: Biased data shapes the content you see (news, ads, social feeds). If the underlying data reflects and amplifies certain viewpoints or characteristics due to selection bias (e.g., volunteer bias in polls, platform-specific data), the digital "reality" presented to you can be significantly skewed, making manipulation through misinformation or targeted messaging easier.
- Ineffective or Manipulative Targeting: Advertising or political campaigns relying on biased data might mistakenly identify entire groups based on the characteristics of an unrepresentative sample. This can lead to alienating messages or, conversely, highly effective but ethically questionable targeting of vulnerable groups whose online behavior data is easily captured due to certain biases (e.g., susceptibility bias).
- Limited Choices and Experiences: Products, services, and user interfaces designed based on data from a non-representative sample might only work well for a specific group, effectively excluding or disadvantaging others. Your digital experience is being "controlled" by data that doesn't reflect the full spectrum of users.
- Reinforcing Inequalities: If data collection systematically underrepresents certain demographics or activities, algorithms trained on this data will struggle to serve those groups well, potentially limiting their access or visibility online and reinforcing existing societal inequalities.
Mitigation: Awareness and Critical Thinking
Addressing selection bias in the digital realm is complex. It cannot usually be fixed simply by applying statistical corrections after the biased data has been collected.
Mitigation: While statistical methods like Heckman correction exist for specific cases, selection bias is often best addressed by careful study design, robust data collection methods aimed at representativeness, transparency about data sources and limitations, and critical evaluation of results.
For data scientists and platform designers, the focus must be on:
- Awareness: Recognizing the potential sources of bias in their specific data pipelines.
- Methodology: Employing sampling techniques that aim for representativeness where possible, or clearly defining the population their data can legitimately represent.
- Transparency: Being upfront about data sources, collection methods, and known limitations or potential biases in their datasets.
For individuals navigating the digital world ("How They Use Data to Control You"):
- Be Critical: Question the data you see. Who collected it? How? Who was included or excluded? Does the claim sound plausible for the entire population, or just a specific group?
- Understand Your Data Footprint: Be aware that the data you generate (clicks, views, purchases, time spent) is being collected and analyzed. This data is a sample of your behavior, filtered by the platforms you use and how you use them, and is subject to selection biases.
- Diversify Information Sources: Counteract algorithmic filter bubbles (a form of observer selection bias) by actively seeking information from diverse perspectives and sources outside of your usual digital channels.
Selection bias is a powerful, often invisible force shaping the digital landscape. By understanding how it works and where it appears, we can become more critical consumers of data-driven insights and more aware of the subtle ways our online experiences and choices can be influenced and potentially controlled by unrepresentative data.
Related Articles
See Also
- "Amazon codewhisperer chat history missing"
- "Amazon codewhisperer keeps freezing mid-response"
- "Amazon codewhisperer keeps logging me out"
- "Amazon codewhisperer not generating code properly"
- "Amazon codewhisperer not loading past responses"
- "Amazon codewhisperer not responding"
- "Amazon codewhisperer not writing full answers"
- "Amazon codewhisperer outputs blank response"
- "Amazon codewhisperer vs amazon codewhisperer comparison"
- "Are ai apps safe"